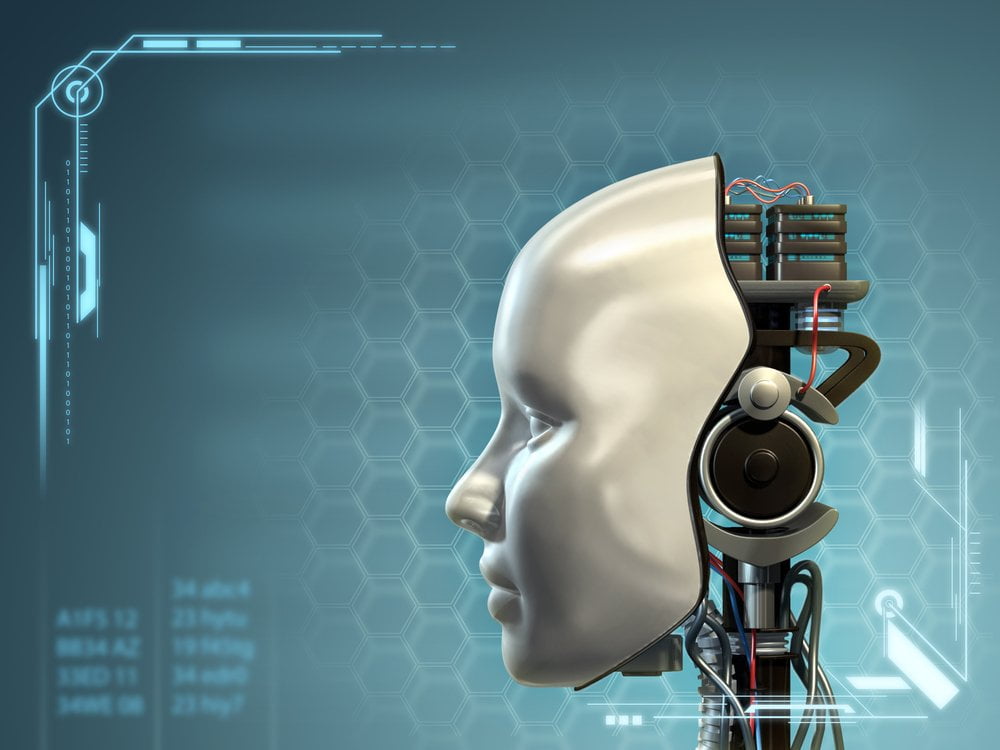
Erik Brynjolfsson, MIT Sloan School professor, explains how rapid advances in machine learning are presenting new opportunities for businesses. He breaks down how the technology works and what it can and can’t do (yet). He also discusses the potential impact of AI on the economy, how workforces will interact with it in the future, and suggests managers start experimenting now. Brynjolfsson is the co-author, with Andrew McAfee, of the HBR Big Idea article, “The Business of Artificial Intelligence.” They’re also the co-authors of the new book, Machine, Platform, Crowd: Harnessing Our Digital Future.
SARAH GREEN CARMICHAEL: Welcome to the HBR IdeaCast from Harvard Business Review. I’m Sarah Green Carmichael.
It’s a pretty sad photo when you look at it. A robot, just over a meter tall and shaped kind of like a pudgy rocket ship, laying on its side in a shallow pool in the courtyard of a Washington, D.C. office building. Workers – human ones – stand around, trying to figure out how to rescue it.
The security robot had just been on the job for a few days when the mishap occurred. One entrepreneur who works in the office complex wrote: “We were promised flying cars. Instead we got suicidal robots.”
For many people online, the snapshot symbolized something about the autonomous future that awaits. Robots are coming, and computers can do all kinds of new work for us. Cars can drive themselves. For some people this is exciting, but there is also clearly fear out there about dystopia. Tesla CEO Elon Musk calls artificial intelligence an existential threat.
But our guest on the show today is cautiously optimistic. He’s been watching how businesses are using artificial intelligence and how advances in machine learning will change how we work. Erik Brynjolfsson teaches at MIT Sloan School and runs the MIT Initiative on the Digital Economy. And he’s the co-author with Andrew McAfee of the new HBR article, “The Business of Artificial Intelligence.”
Erik, thanks for talking with the HBR IdeaCast.
ERIK BRYNJOLFSSON: It’s a pleasure.
SARAH GREEN CARMICHAEL: Why are you cautiously optimistic about the future of AI?
ERIK BRYNJOLFSON: Well actually that story you told about the robot that had trouble was a great lead in because in many ways it epitomizes some of the strengths and weaknesses of robots today. Machines are quite powerful and in many ways, they’re superhuman you know just as a calculator can do arithmetic a lot better than me, we’re having artificial intelligence that’s able to do all sorts of functions in terms of recognizing different kinds of cancer images, or now getting superhuman even in speech recognition in some applications but they’re also quite narrow. They don’t have general intelligence the way people do. And that’s why partnerships of humans and machines are often going to be the most successful in business.
SARAH GREEN CARMICHAEL: You know it’s funny, cause when you talk about image recognition I think about a fantastic image in your article that is called “Puppy or Muffin.” I was amazed at how much puppies and muffins look alike in sort of even more amazed that robots can tell them apart.
ERIK BRYNJOLFSSON: Yeah, it’s a funny image. It always gets a laugh and encourage people to go take a look at it. And there are lots of things that humans are pretty good at in distinguishing different kinds of images. And for a long time, machines were nowhere near as good as recently as seven, eight years ago, machines made about a 30 percent error rate on image net, this big database that Fei Fei Li created of over 10 million images. Now machines are down less, you know, less than 5%, 3-4% depending on how it’s set up. Humans still have about a 5% error rate. Sometimes they get those puppies and nothing’s wrong. Be careful what you reach for next time you’re at that breakfast bar. But that’s a good example.
The reason it’s improved so much in the past few years is because of this new approach using deep neural nets that’s gotten much more powerful for image recognition and really all sorts of different applications. I think that’s a big reason why there’s so much excitement these days.
SARAH GREEN CARMICHAEL: Yeah, it’s one of those things where we all kind of like to make fun of machines that get it wrong but also it’s sort of terrifying when they get it right.
ERIK BRYNJOLFSSON: Yeah. Machines are not going to be perfect drivers, they’re not going to be perfect at making credit decisions that are going to be perfect at distinguishing you know muffins and puppies. And so, we have to make sure we build systems that are robust to those imperfections. But the point we make an article, Andy and I point out that you know humans aren’t perfect at any of those tasks either. And so, the benchmark for most entrepreneurs and managers is: who’s going to be better for solving this particular task or better yet can we create a system that combines the strengths of both humans and machines and does something better than either of them would do individually.
SARAH GREEN CARMICHAEL: With photo recognition and facial recognition, I know that Facebook facial recognition software can’t tell the difference between me wearing makeup and me not wearing makeup, which is also sort of funny and horrifying right? But at the same time, you know, I think a lot of us struggle to recognize people out of context, we see someone at the grocery store and we think you know, I know that person from somewhere. So, it’s something that humans don’t always get right either.
ERIK BRYNJOLFSSON: Oh yeah. I’m the world’s worst. You know at conferences I would love it if there was a little machine whispering in my ear who this person is and how I met them before. So there, you know, there are those kinds of tradeoffs. But it can lead to some risks. For instance, you know if machines are making bad decisions on important things, like who should get parole or who gets credit or not. That could be really problematic. Worse yet, sometimes they have biases that are built in from the data sets they use. If the people you hire in the past all had a certain kind of ethnic or gender tilt to them, then if you use a training set and teach the machine how to hire people it will learn the same biases that the humans had previously. And, of course, that can be perpetuated and scaled up in ways that we wouldn’t like to see.
SARAH GREEN CARMICHAEL: There is a lot of hype right now around AI or artificial intelligence. Some people say machine learning, other people come along and say: hold on hold on hold on, like a lot of this is just software and we’ve been using it for a long time. So how do you kind of think through the different terms and what they really mean?
ERIK BRYNJOLFSSON: Well there’s a really important difference between the way the machines are working now versus previously you know any — McAfee and I wrote this book The Second Machine Age where we talked about having machines do more and more cognitive tasks. And for most of the past 30 or 40 years that’s been done by us painstakingly programming, writing code of exactly what we want the machine to do. You know if it’s doing tax preparation, add up this number and multiply it by that number, and of course we had to understand exactly what the task was in order to specify it.
But now the new machine learning approaches literally have the machines learn on their own things that we don’t know how to explain — the face recognition is a perfect example. It would be really hard for me to describe you know my mother’s face, you know how far apart are her eyes or what does her ear look like.
ERIK BRYNJOLFSSON: I can recognize it but I couldn’t really write code to do it. And the way the machines are working now is, instead of having us write the code, we give them lots and lots of examples. You know here are pictures of my mom from different perspectives, or here pictures of cats and dogs or here’s a piece of speech you know with the word “yes” and the word “no.” And if you give them enough examples the machine learning algorithms figure out the rules on their own.
That’s a real breakthrough. It overcomes what we call Polanyi’s paradox. Michael Polanyi the Polymath and philosopher from the 1960s famously said “We all know more than we can tell but with machine learning we don’t have to be able to tell or explain what to do. We just have to show examples.” That change is what’s opening up so many new applications for machines and allowing it to do a whole set of things that previously only humans could do.
SARAH GREEN CARMICHAEL: So, it’s interesting to think about kind of the human work that has to just go into training the machines like someone who would sit there literally looking at pictures of blueberry muffins and tagging them “muffin, muffin, muffin” so the machine you know learns that’s not a Chihuahua, that’s a blueberry muffin. Is that the kind of thing where in the future you could see that kind of rote algorithm, machine training work being kind of a low-paid dead-end job whereas maybe that person once would have had a more interesting job but now the machine has the more interesting job.
ERIK BRYNJOLFSSON: I don’t think that’s going to be a big source of employment, but it is true there are places like Amazon’s Mechanical Turk where thousands of people do exactly what you said, they tag images and label them. That’s how ImageNet the database of millions of images got labeled. And so, there are people being hired to do that. Companies sometimes find that training machines by having humans tagged the data is one way to proceed.
But often they can find ways of having data that’s already tagged in some way, that’s generated from their enterprise resource planning system or from their call center. And if they’re clever, that will lead to the creation of this tag data, and I should back up a bit and say that machines, one of their big weaknesses is that they really do need tag data. That’s the most powerful kind of algorithm, sometimes called supervised learning, where humans have the advanced tag and explained what the data means.
And then the machine learns from those examples and eventually can extrapolate it to other kinds of examples. But unlike humans, they often need thousands or even millions of examples to do a good job whereas you know, a two-year-old probably would learn after one or two times what a cat was versus a dog was that you wouldn’t have to show, you know, 10,000 pictures of a cat before they got it.
SARAH GREEN CARMICHAEL: Right. Given where we are with AI and machine learning right now, on balance, do you feel like this is something that is overhyped and people talk too much about sort of too science fiction terms or is it something that’s not quite hyped enough and actually people are underestimating what it could do in the relatively near future.
ERIK BRYNJOLFSSON: Well it’s actually both at the same time, if you can believe it. I think that people have unrealistic expectations about machines having all these general capabilities kind of from watching science fiction like the Terminator. And if a machine can understand Chinese characters you might think it also could understand Chinese speech and it could recommend a good Chinese restaurant, know a little bit about the Xing dynasty and none of that would be true. A machine that can play expert chess can’t even play checkers or go or other games. So, in a way they’re very narrow and fragile.
But on the other hand, I think the set of applications for those narrow capabilities is quite large, using that supervised learning algorithms, I think there are a lot more specific tasks that could be done that we’ve only scratched the surface of and because they’ve improved so much in the past five or 10 years, most of those opportunities have not yet really been explored or even discovered yet. There’s a few places where the big giants like Google and Microsoft and Facebook have made rapid progress, but I think that there are literally tens of thousands of more narrow applications that small and medium businesses could start using machine learning for in their own areas.
SARAH GREEN CARMICHAEL: What are some examples of ways that companies are using this technology right now?
ERIK BRYNJOLFSSON: Well one of my favorite ones I learned from my friend Sebastian Thrun He’s the founder of Udacity, the online learning course, which by the way is a good way to learn more about these technologies. But he found that when people were coming to his site and asking questions on the chat room, some of the sales people were doing a really good job of come to the right course and closing the sale and others, well, not so much. This created a set of training data.
He and his grad student realized that if they took the transcripts they would see that certain sets of words in certain dialogues lead to success and sales and others didn’t. And he fed that information into a machine learning algorithm and it started identifying which patterns of phrases and answers were the most successful.
But what happened next was I think especially interesting instead of just trying to build a bot that would answer all the questions, they built a bot that would advise the human salespeople. So now when people go to the site the bot kind of looks over the shoulder of the human and when it sees some of those key words it whispers into his or her ear: “hey, you know you might want to try this phrase or you might want to point him to this particular course.”
And that works well for the most common kinds of queries, but the more obscure ones that the bot has never seen before the human is much better at. And this kind of partnership is a great example of an effective use of AI and also how you can use existing data to turn into a tag data set that the supervised learning system benefits from.
SARAH GREEN CARMICHAEL: So how did these people feel about being coached by a bot?
ERIK BRYNJOLFSSON: Well, it’s helped them close their sales so it’s made them more productive. Sebastian says it’s about 50% more successful when they’re using the bot. So I think it’s been it’s been beneficial in helping them learn more rapidly than they would have if they just kind of stumbled all along.
Going forward, I think this is an example of how the bots are often good at the more routine repetitive kinds of tasks, the machines can do the ones that they have lots of data for. And the humans tend to excel at the more unusual tasks for most of us. I think that’s kind of a good trade-off. Most of us would prefer having kind of more interest in varied work lives rather than doing the same thing over and over.
SARAH GREEN CARMICHAEL: So, sales is a form of knowledge work right and you sort of gave an example there. One of the big challenges in that kind of work is that you can’t — it’s really hard to scale up one person’s productivity if you are a law firm, for example, and you want to serve more clients have to hire more lawyers. It sounds like AI could be one way to get finally around that conundrum.
ERIK BRYNJOLFSSON: Yeah AI certainly can be a big force multiplier. It’s a great way of taking some of your best, you know, lawyers or doctors and having them explain how they go about doing things and give examples of successes and the machine can learn from those and replicate it or be combined with people who are already doing the jobs and help in a way coached them or handle some of the cases that are most common.
SARAH GREEN CARMICHAEL: So, is it just about being more productive or did you see other examples of human machine collaboration that tackled different types of business challenges?
ERIK BRYNJOLFSSON: Well in some cases it’s a matter of being more productive, in many cases, a matter of doing the job better than you could before. So there are systems now that can help read medical images and diagnose cancer quite well, the best ones often are still combined with humans because the machines make different kinds of mistakes in the humans so that the machine often will create what are called false positives where it thinks there’s cancer but it’s really not and the humans are better at ruling those out. You know maybe there was an eyelash on the image or something that was getting in the way.
And so, by having the machine first filter through all the images and say hey here are the ones that look really troubling. And then having a human look at those ones and focus more closely on the ones that are problematic, you end up getting much better outcomes than if that person had to look at all the images herself or himself and maybe, maybe overlook some potentially troubling cases.
SARAH GREEN CARMICHAEL: Why now? Because people predicted for a long time that I was just around the corner and sounds like it’s finally starting to happen and really make its way into businesses. Why are we seeing this finally start to happen right now?
ERIK BRYNJOLFSSON: Yes, that’s a great question. It’s really the combination of three forces that have come together. The first one is simply that we have much better computer power than we did before. So, Moore’s Law, the doubling of computer power is part of it. There’s also specialized chips called GPUs and TPUs that are another tenfold or even a hundredfold faster than ordinary chips. As a result, training a system that might have taken a century or more if you done it with 1990s computers can be done in a few days today.
And so obviously that opens up a whole new set of possibilities that just wouldn’t have been practical before. The second big force is the explosion of digital data. Data is the lifeblood of these systems, you need them to train. And now we have so many more digital images, digital transcripts, digital data from factory gauges and keeping track of information, and that all can be fed into these systems to train them.
And as I said earlier, they need lots and lots of examples. And now we have digital examples in a way we didn’t previously and in the end with the Internet are things you can imagine it’s going to be a lot more digital data going forward. And last but not least, there have been some significant improvements in the algorithms the men and women working in these fields have improved on the basic algorithms. Some of them were first developed literally 30 years ago, but they’ve now been tweaked and improved, and by having faster computers and more data you can learn more rapidly what works and what doesn’t work. When you put these three things together, computer power, more data, and better algorithms, you get sometimes as much as a millionfold improvement on some applications, for instance recognizing pedestrians as they cross the street, which of course is really important for applications like self-driving cars.
SARAH GREEN CARMICHAEL: If those are sort of the factors that are pushing us forward, what are some of the factors that might be inhibiting progress?
ERIK BRYNJOLFSSON: What’s not holding us back is the technology, what is holding us back is the imagination of business executives to use these new tools in their businesses. You know, with every general-purpose technology, whether it’s electricity or the internal combustion engine the real power comes from thinking of new ways of organizing your factory, new ways of connecting to your customers, new business models. That’s where the real value comes. And one of the reasons we were so happy to write for Harvard Business Review was to reach out to people and help them be more creative about using these tools to change the way they do business. That’s where the real value is.
SARAH GREEN CARMICHAEL: I feel like so much of the broader conversation that AI is about, will this create jobs or destroy jobs? And I’m just wondering is that a question that you get asked a lot, and are you sick of answering it?
ERIK BRYNJOLFSSON: Well of course it gets asked a lot. And I’m not sick of answering because it’s really important. I think the biggest challenge for our society over the next 10 years is going to be, how are we going to handle the economic implications of these new technologies. And you introduced me in the beginning as a cautious optimist, I think you said, and I think that’s about right. I think that if we handle this well this can and should be the best thing that ever happened to humanity.
But I don’t think it’s automatic. I’m cautious about that. It’s entirely possible for us to not invest in the kind of education and retraining of people to not do the kinds of new policies, to encourage business formation and new business models even. Income distribution has to be rethought and tax policy things like the earned income tax credit in the United States and similar wage subsidies in other countries.
ERIK BRYNJOLFSSON: We need to make a bunch of changes across the board at the policy level. Businesses need to rethink how they work. Individuals need to take personal responsibility for learning the new skills that are going to be needed going forward. If we do all those things I’m pretty optimistic.
But I wouldn’t want people to become complacent, because already over the past 10 years a lot of people have been left behind by the digital revolution that we’ve had so far. And looking forward, I’d say we ain’t seen nothing yet. We have incredibly powerful technologies especially in artificial intelligence that are opening up new possibilities. But I want us to think about how we can use technology to create shared prosperity for the many, not just the few.
SARAH GREEN CARMICHAEL: Are there tasks or jobs that machine learning, in your opinion, can’t do or won’t do?
ERIK BRYNJOLFSSON: Oh, there are so many. Just to be totally clear, most things, machine learning can’t do. It’s able to do a few narrow areas really, really well. Just like a calculator can do a few things really, really well, but humans are much more general, much more broad set of skills, and the set of skills that humans can do it is being encroached on.
Machines are taking over more and more tasks are combining, teaming up in more and more tasks but in particular, machines are not very good at very broad-scale creativity you know. Being an entrepreneur or writing a novel or developing a new scientific theory or approach, those kinds of creativity are beyond what machines can do today by and large.
Secondly, and perhaps for an even broader impact, is interpersonal skills, connecting with the humans. You know we’re wired to trust and care for it and be interested in other humans in a way that we aren’t with other machines.
So, whether it’s coaching or sales or negotiation or caring for people, persuading, people those are all areas where humans have an edge. And I think there will be an explosion of new jobs whether it’s for personal coaches or trainers or team oriented activities. I would love to see more people learning those kinds of softer skills that machines are not good at. That’s where they’ll be a lot of jobs in the future.
SARAH GREEN CARMICHAEL: I was surprised to see in the article though, that some of these AI programs are actually surprisingly good at recognizing human emotions. I was really startled by that.
ERIK BRYNJOLFSSON: I have to be careful. One of the main things I learned working with Andy and going to visit all these places is never say never, any particular thing that one of us said “oh this will never happen,” you know, we find out that someone is working in a lab.
So my advice is that their relative strengths and relative weaknesses and emotional intelligence, I still think is a relative strength of humans, but there are particular narrow applications where machines are improving quite rapidly. Affectiva, a company here in Boston has gotten very good at reading emotions, is part of what you need to do to be a good coach to be a caring person, is not the whole picture, but it is one piece of the interpersonal skills that machines are helping with.
SARAH GREEN CARMICHAEL: What do you see as the biggest risks with AI?
ERIK BRYNJOLFSSON: I think there are a few. One of the big risks is that these machine learning algorithms can have implicit biases and they can be very hard to detect or correct. If the training data is biased, has some kind of racial or ethnic or other biases in its data, then those can be perpetuated in the sample. And so, we need to be very careful about how we train the systems and what data we give them.
And it’s especially important because they don’t have the kind of explicit rules that earlier waves of technology had. So, it’s hard to even know. It’s unlikely to have a rule that says, you know, don’t give loans to black people or whatever, but it may implicitly have its thumb on the scale in one way or the other if the training data were biased.
SARAH GREEN CARMICHAEL: Right. Because it might notice for instance that, statistically speaking, black people get turned down more for loans that kind of thing.
ERIK BRYNJOLFSSON: Yeah, if the people who you had made those decisions before were biased in a use for the training data that could end up creating a biased training set. And you know, maybe nobody explicitly says that they were biased, but it sort of shows up in other subtle ways based on the, you know, the zip code that someone’s coming from or their last name or their first name or whatever. So that would be subtle things that you need to be careful of.
The other thing is what we touched on earlier just the whole, what’s happening with income inequality and opportunity as the machines get better at many kinds of tasks, you know, driving a truck or handling a call center. The people who had been doing those jobs need to find new things to do. And often those new jobs won’t be paying as well if we aren’t careful. So that could be a real income hit. Already we see growing income inequality.
We have to be aggressive about thinking how we can create broadly shared prosperity. One of the things we did at MIT is we launched something called the Inclusive Innovation Challenge which recognizes and rewards organizations that are using technology to create shared prosperity, they’re innovating in ways that do that. I’d love to see more and more entrepreneurs think in that way not just how they can create concentrated wealth, but how they can create broadly shared prosperity.
SARAH GREEN CARMICHAEL: Elon Musk has been out there saying artificial intelligence could be an existential threat to human beings. Other people have talked about fears that the machines could take over and turn against us. How do you feel about those kinds of concerns?
ERIK BRYNJOLFSSON: Well, like I said earlier, you can never say never and, you know, as machines kept getting more and more powerful I can imagine them having enormous powers especially as we delegate more of the operations of our critical infrastructure in our electricity and our water system and our air traffic control and even our military operations to them. But the reason I didn’t list it is I don’t see it as the most immediate risk right now, the technologies that are being rolled out right now, they have effects on bias and decision making their effect on jobs and income. But by and large they don’t have those kinds of existential risks.
I think it’s important that we have researchers working in those areas and thinking about them but I wouldn’t want to, to panic Congress or the people right now into doing something that would probably be counterproductive if we overreacted right now.
I think it’s an area for research but in terms of devoting billions of dollars of effort, I would put that towards education and retraining and handling bias — the things that are facing us right now and will be facing us for the next five and 10 years.
SARAH GREEN CARMICHAEL: What do you feel is the appropriate role of regulation as AI develops?
ERIK BRYNJOLFSSON: I think we need to be watchful, because there’s the potential for AI to lead to more concentration of power and more concentration of wealth. The best antidote to that is competition.
And what we’ve seen the tech industries, for most of the past 10, 20, 30 years is that as one monopolist, whether it’s IBM or Microsoft, gets a lot of power, another company comes along and knocks it off its perch. I remember teaching a class where about 15 years ago a speaker said you know Yahoo has search locked up no one’s ever going to displace Yahoo. So you know we need to be humble and realize that the giants of today face threats and could be overturned.
That said, if there becomes a sort of a stagnant loss of innovation and these companies have a stranglehold on markets and maybe have other adverse effects in areas like privacy, then it would be right for government to step in. My instinct right now would be sort of watchful waiting, keeping an eye on these companies and doing what we could to foster innovation and competition as the best way to protect consumers.
SARAH GREEN CARMICHAEL: So, if all of this still sounds quite futuristic to the average manager, if they’re kind of like: OK, you know this is sort of way outside of what I’m working on in my role, what are the sort of things that you’d advise people to keep in mind or think about?
ERIK BRYNJOLFSSON: Well it starts with realizing this is not futuristic and way out there. There are lots of small and medium sized companies that are learning how to apply this right now, whether it’s, you know, sorting cucumbers to be more effective, somebody wrote an application that did that, to helping with recommendations online. There’s a company I’m advising called Infinite Analytics that is giving customers better recommendations about what products they should be choosing, to helping with, you know, credit decisions.
There are so many areas where you can apply these technologies right now you can take courses or you can have people in your organization take courses or you can hire people at places like Udacity or fast.ai, my friend Jeremy Howard runs a great course in that area, and put it to work right away and start with something small and simple.
But definitely don’t think of this as futuristic. Don’t be put off by the science fiction movies whether, you know, the Terminator or other AI shows. That’s not what’s going on. It’s a bunch of very specific practical applications that are completely feasible in 2017.
SARAH GREEN CARMICHAEL: Erik, thanks so much for talking with us today about all of this.
ERIK BRYNJOLFSSON: It’s been a real pleasure.
SARAH GREEN CARMICHAEL: That’s Erik Brynjolfsson. He’s the director of the MIT Initiative on the Digital Economy. And he’s the co-author with Andrew McAfee of the new HBR article, ” The Business of Artificial Intelligence.”
You can read their HBR article, and also read about how Facebook uses AI and Machine learning in almost everything you see, and you can watch a video – shot in my own kitchen! – about how IBM’s Watson uses AI to create new recipes. That’s all at hbr.org/AI.
Thanks for listening to the HBR IdeaCast. I’m Sarah Green Carmichael.
[“Source-hbr”]